Algae Storm
Addressing the challenge of cyanobacterial blooms, SMHI integrates AI with Sentinel-3 satellite data to develop early warning systems for the Baltic Sea.
In a nutshell
- Cyanobacterial blooms in the Baltic Sea disrupt ecosystems and human activities, posing risks to health and marine biodiversity.
- SMHI leverages AI and Sentinel-3 satellite data to develop an advanced early warning system for algal blooms.
- Improved bloom predictions will support climate resilience, ecosystem protection, and early public warnings.
Technical Overview
Challenge
Phytoplankton is the foundation of the ocean’s food ecosystem. When they grow rapidly, the term algal bloom is used. Some algal blooms are problematic and can disrupt tourism, aquaculture, marine plants and animals. In addition there are algal toxins that can harm human health.
The algal bloom season in the seas begins in the spring when sunlight is more readily available. Phytoplankton, such as diatoms, grow rapidly, leading to the consumption of nutrients like nitrate and phosphate. In the Baltic Sea, nitrate is depleted in the surface water while phosphate remains after the spring bloom. This favors the growth of cyanobacteria, which can use dissolved nitrogen gas as a nitrogen source, allowing them to outcompete other phytoplankton. The biomass of cyanobacteria builds up over time. In the summer between June and September the algae often accumulate at the surface becoming visible to the eye. This typically happens when there are weak winds and is commonly referred to as algal blooms.
To better predict the development and direction of algal blooms, a ten-week pilot study was undertaken by Research Institutes of Sweden (RISE) and the Swedish Meteorological and Hydrological Institute (SMHI). The pilot study investigated how artificial intelligence and satellite observations can contribute to providing earlier forecasts to warn the public.
DestinE Solution

SMHI are experts in oceanography and have long used satellite observations of algal blooms, water temperature, and more. Now, observations and results from oceanographic models will be used together with artificial intelligence (AI) to improve algal forecasts. The goal is to develop a system for SMHI to provide early warnings for cyanobacterial blooms in the Baltic Sea. To achieve this, the project team has investigated how AI can be utilized in combination with satellite images from the Sentinel-3 Ocean Land Colour Instrument (OLCI) sensor.
In practice, several steps need to be undertaken to be able to predict the activity of cyanobacteria in the Baltic Sea, these are outlined in figure 1. As a first step a suitable time series dataset was selected to train the AI model on. In our case, we limited the scope to only include Sentinel-3 OLCI Level 2 Ocean Colour Full Resolution data, but in theory more data sources could be combined to train the model. The next step is to perform pre-processing of the dataset, making sure that the dataset covers only the Baltic Sea, and has a suitable resolution and projection. Once pre-processing is performed we upload the dataset to a s3 container hosted on the DEDL Islet service. We then proceed with preparing the dataset for modelling, followed by training the model. For our use-case the model architecture follows a classic autoencoder (encoder-decoder) neural network, with the encoder consisting of two 2D convolutional layers, and the decoder mirroring this structure in reverse. The idea behind this choice comes from the assumption that changes in algae levels between consecutive days are gradual, making today’s algae levels a strong predictor for estimating those of the next day. The output is a prediction of the pixel value from a certain band. We then classify each as indicating algae bloom or not according to some threshold values.
All the pre-processing of data and modelling was performed on the JupyterLab hub provided on the Destination Earth Data Lake (DEDL) Stack service. Storage of satellite imagery was done on S3 provided by the DEDL Islet service.
Impact
The model architecture and first results show promise that prediction of algae bloom on pixel level is possible. Based on the learnings from the project it is reasonable to believe that we can improve on the forecasts by including more datasets from Destination Earth Data Lake and by further developing the Deep Learning system discussed earlier. An interesting avenue for investigation here is the inclusion of digital twin datasets to improve on the model, but also the potential for algae bloom predictions to be included in a Digital Twin.
A performant Deep Learning system for cyanobacteria bloom would in the long term facilitate us to work better with different scenarios in a changing climate, improving on SMHIs Baltic Algae Watch System and potentially inspire for similar systems at other locations than the Baltic Sea. In the long term, this could help provide earlier warnings to the public of algae blooms.
Contributions
Providers
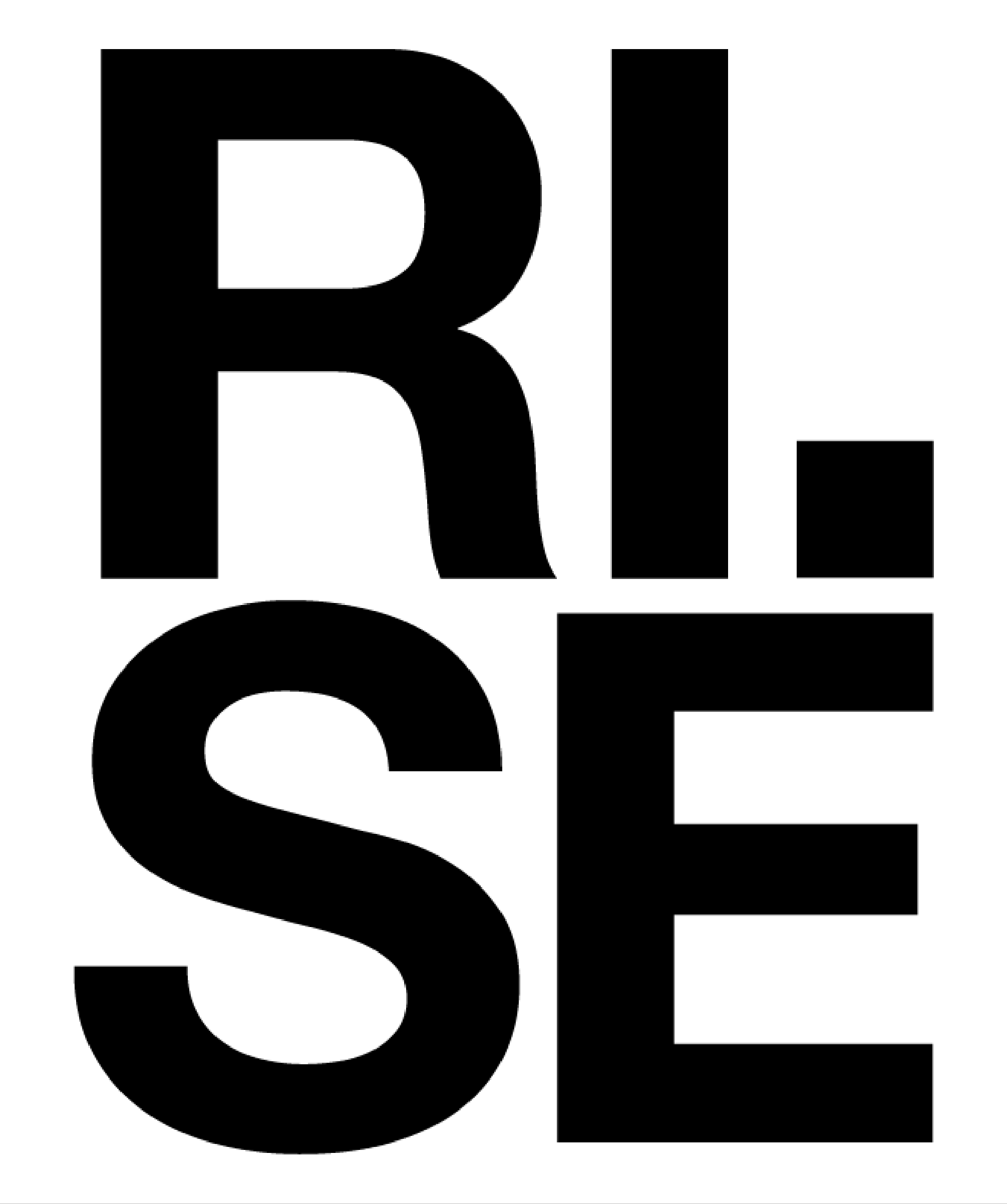
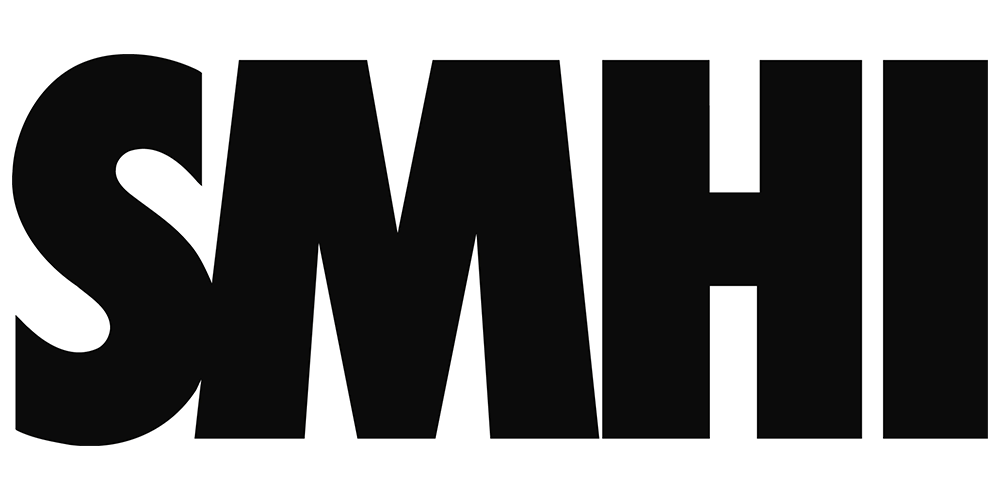